ARRIS
A common challenge theme among future particle physics experiments is the need to process, efficiently, intelligently, precisely, and adaptively, high rates of continuous data generated by high-resolution imaging devices. A prime example of detector technology facing this challenge is that of Liquid Argon Time Projection Chambers (LArTPCs). This technology is widely used for neutrino and dark matter particle detection, and it is being aggressively pursued and being scaled up in orders of magnitude for future experiments over the coming decade. This detector technology is unique, in that it operates as a high-resolution stereoscopic imaging device, generating up to several terabytes of high resolution images per second, which must be carefully sifted and examined to look for rare physics signals of interest. Such signals are typically expected to be faint, and not easily distinguishable from ambient background, from a visual perspective. Yet, the image-functioning principle of these detectors is ideally suited for the application of intelligent image processing techniques that could be adapted and integrated in the readout and data acquisition systems of these future-generation detectors. Image processing techniques using deep learning have proliferated in recent years, and even within the LArTPC community, Deep Neural Networks (DNNs), for example, have been demonstrated through simulation studies for their excellent ability to precisely examine LArTPC data, often outperforming traditional hit-based reconstruction methods.
This project builds on the collaboration between physicists and computer scientists at Columbia University targeting hardware acceleration of intelligent algorithms, such as DNNs, for processing particle-trace imagery at scale, and in real time. While GPUs have been proven effective for accelerating the training DNNs for such applications, FPGAs are emerging as an interesting alternative for DNN inference acceleration thanks to their configurability, scalability, parallelism, and power-efficiency. The ultimate goal is the development of transformative ideas and technology that lend themselves to the design of future readout and data acquisition systems, made possible through the close collaboration between physicists and computer scientists, with the latter bringing in expertise in FPGA design and providing insights on hardware acceleration of complex computational algorithms.
Collaborators Include:
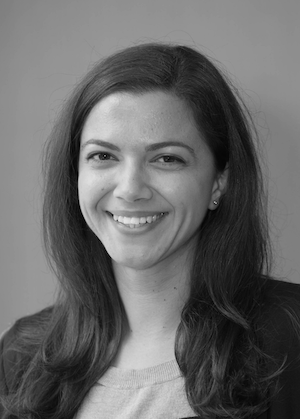
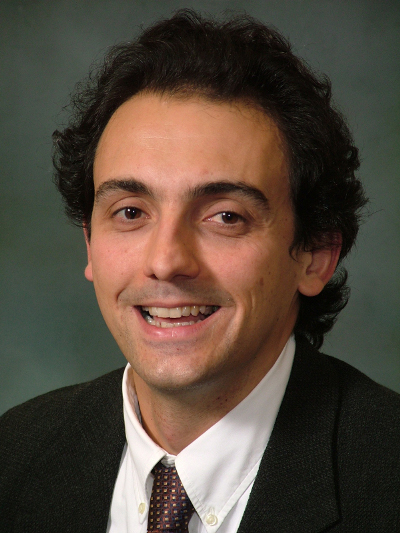
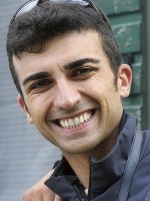
Presentations
- 10 Sep 2019 - "(Fast) Machine Learning for Neutrinos", Georgia Karagiorgi, Fast Machine Learning Workshop
- 13 Jun 2019 - "Accelerating Deep Neural Networks For Real-time Data Selection For High-resolution Imaging Particle Detectors", Georgia Karagiorgi, New York Scientific Data Summit
Publications
- Accelerating Deep Neural Networks for Real-time Data Selection for High-resolution Imaging Particle Detectors, Y. Jwa, G. Guglielmo, L. Carloni and G. Karagiorgi, Unknown (01 Jun 2019) [1 citation].